AI, the Algorithmic Bias and the Skewed Gender Dynamics: How to Drive Gender Equality in AI
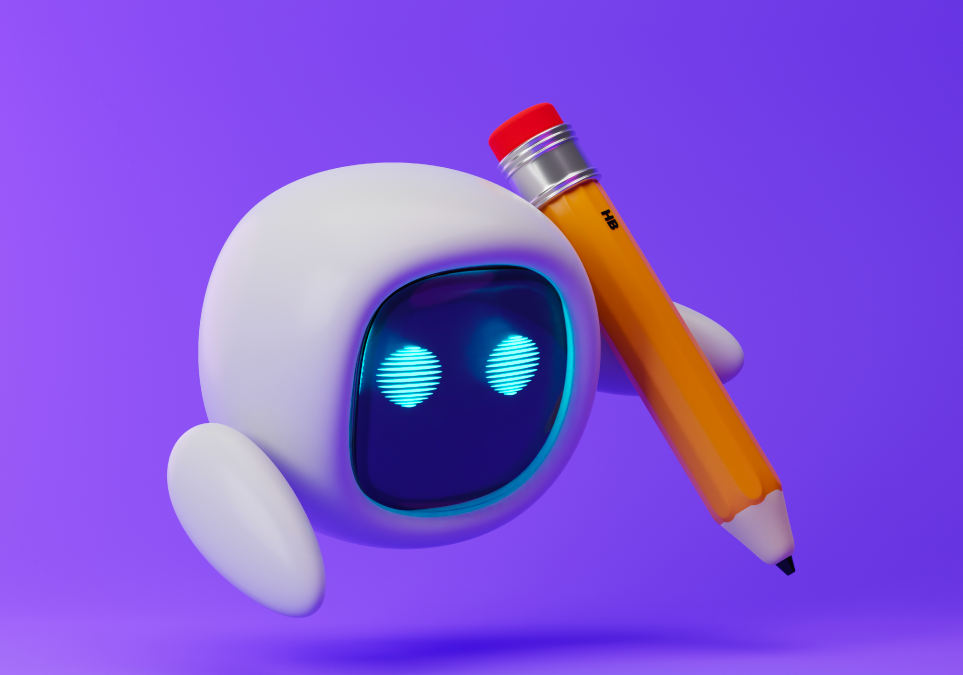
As a woman working in the field of data science and artificial intelligence (AI), I’d like to, first of all, acknowledge the essence and power of women to achieve and thrive in the global ecosystem. That said, one thing I’d like to reflect on is the role of AI in accelerating the gender gap closure, and in giving all females equal opportunities.
Artificial intelligence is still in its nascent stage, but it continues to affect our daily lives in several different ways -- job recruitment, approval of bank loans, search engines, and criminal sentencing to name a few. While AI governs a relatively small part of our lives in the present, it is set to play an extremely important role in every aspect of our daily lives. Moreover, high hopes have been placed on artificial intelligence to address the gender equality question. Hence, before we go into the hows and whens of it, it is critical to understand how AI could affect our lives and its potential contribution to gender equality.
Like all cutting-edge, high-growth domains, artificial intelligence (AI) is not without its challenges. And I argue that the most widespread is the challenge posed by gender inequality.
A report published by the AI Now Institute (April 2019) found that only 18% of authors at leading AI conferences are women and more than 80% of professors in the field are men. At market-leading companies, the situation is even more confronting. Only 15% of AI researchers at Facebook and 10% of AI researchers at Google are women.
Similar “diversity disaster” numbers (as the report calls them) are further highlighted in the World Economic Forum’s Global Gender Gap Report for 2021. Women only make up 14% of cloud computing jobs and 32% of data and AI positions. And only 10.4% of women are pursuing positions in STEM (compared with over 30% of men). Additionally, women only make up 42% of higher-level positions despite being almost half of the workforce, a number that is surely lower if you were to sample only STEM positions.
Gender Bias in AI
These numbers signal that AI systems are at the risk of inadvertently replicating the biases. After all, an AI system is what it eats – the data fed by its creators. If development teams share the same male perspective, then the tools they create will, at best, be limited and, at worst, extend gender discrimination into the digital arena.
Many people assume that because algorithms process information using computational logic, they cannot behave in a discriminatory manner. Indeed, one of the most persuasive arguments for the potential of AI lies in the fact that, if implemented correctly, it could iron out human bias from vital decision-making processes. However, the reality is not so straightforward. AI works by recognizing patterns in vast datasets that would otherwise be too unwieldy for human analysis.
This does, however, mean that discriminatory logic can end up wired into the functioning of AI if the system is insensitive to pre-existing biases in the data. This, in turn, would lead to an AI application making biased decisions regarding certain groups of people, particularly, women.
The Exposé
This flaw was painfully exposed at one of the biggest technology companies when Amazon employed an experimental AI-driven hiring tool in order to efficiently review CVs. It was later found out that the hiring algorithm was systematically discriminating against female candidates.
However, the problem of gender bias isn’t confined to pre-existing issues contained within historical data. All-male developer teams are liable to inadvertently create ineffective AI on account of their failure to pre-empt how their algorithms might produce discriminatory outcomes.
If only certain groups of people build the technology then it is highly likely that discrimination is inevitable, even if the discrimination is not intentional. In her book “Invisible Women: Exposing Data Bias in a World Designed for Men”, journalist and activist Caroline Criado Perez makes a compelling case that data is actually far from objective and is often highly male-biased. As a result of this bias, decisions around public spending, health, education, the workplace and society in general suffer.
In essence, a whole host of structural factors aggregate to make a career in AI far more attractive to men than women in general.
How can we bring about the change?
I wish there was a magic bullet that would fix all this but I know that change only happens as a result of a concerted, joint effort and activities focused on consciously making this change happen.
Consequently, real progress can only come if industry stakeholders commit to a multi-faceted strategy that addresses the causes of gender inequality from a number of angles.
At ZestMoney, we make consistent and concerted efforts to ensure that there is no bias in our proprietary algorithms to scale financial inclusion for women. We are building a digital solution that allows us to collect some behavioral and knowledge-based inputs from women borrowers – like their family liabilities, understanding of their finances, their expenses, mobile usage data, and level of their general, and financial literacy – to build a women-specific robust scoring model that can support lending to more women.
We also ensure that our data science, product and technology teams have a healthy balance of gender representation which facilitates a diversity of opinions, solutions and approaches.
How to accelerate the change and what can be done to make this proportion more equal/balanced?
According to the 2021 AI Index, men currently make up about 70% of the applicant pool for AI jobs.
The first step is to expand the available talent pool by increasing the number of female applicants for data science and developer roles.
The technology industry can certainly play its part in supporting female STEM graduates to pursue careers in technology and in doing more to ensure women do not leave these careers prematurely with initiatives focused on child care, work-life balance and more funding available for female entrepreneurs. However, where companies can make the most immediate impact is by internally transforming so that their workplace is more accommodative of driven and technologically proficient women.
There’s never been a more exciting time to be part of the (financial) technology industry. This not only means a fantastic opportunity to build cutting-edge technology but also to change perceptions of the industry and ultimately ensure that AI becomes an engine for positive change.
Since the problem has been identified early, corrective steps will yield results only with collective efforts both at an individual and company level. If we can do that, I have no doubt that we can foster an AI ecosystem towards a more inclusive and gender-balanced, which in turn will contribute to building fair and less prone to biased AI solutions.